IN TODAY'S SIGNAL
|
|
Read Time: 5 min 27 sec
|
|
|
|
Enjoying this newsletter? Please forward it to a friend or colleague. It helps us keep this content free.
|
|
|
|
BREAKTHROUGH
|
Language Models
|
OpenAI Releases GPT-4o: Text, Vision, Audio In a Single Record-Breaking Model and Desktop App |
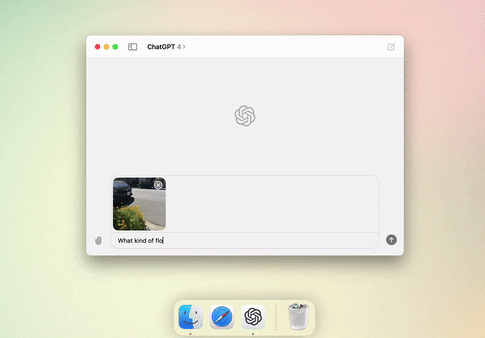 |
What's New |
OpenAI just demonstrated the capabilities of their newest flagship model GPT-4o, available through a new updated UI, and also through the API.
GPT-4o - the o stands for "Omnimodel" - is a conversational model that improves on its capabilities over text, audio and vision. Unlike previous approaches combining Intelligence, Text-to-Speech and Audio model, this new model reasons across voice, text and vision.
This makes GPT-4o way faster, but also more conversational and natural. It can understand the context and the tone of the conversation, and can answer using a variety of tones as well. It can also be interrupted, which improves the experience and makes it all the more natural.
GPT-4o can be combined with: real-time vision (images and videos), memory, GPTs, browse, and advanced data analysis.
More importantly, GPT-4o is:
available through the UI and the API
2x faster
50% cheaper
5x higher rate limits compared to GPT-4 turbo
available to all users for free
The latency is the real game-changer here with improved speeds in over 50 different languages. This is the smartest and most natural assistant ever showcased live by OpenAI.
For example, during the live demonstration, GPT-4o assisted someone in solving a linear equation step-by-step while they filmed themselves working on the paper.
OpenAI also rolled out the desktop version of ChatGPT, and an improved Web UI to make the user experience even more seamless.
Lastly, OpenAI also shared their continuous work regarding the Safety of their models, and highlighted the new challenges that real-time audio and vision bring.
|
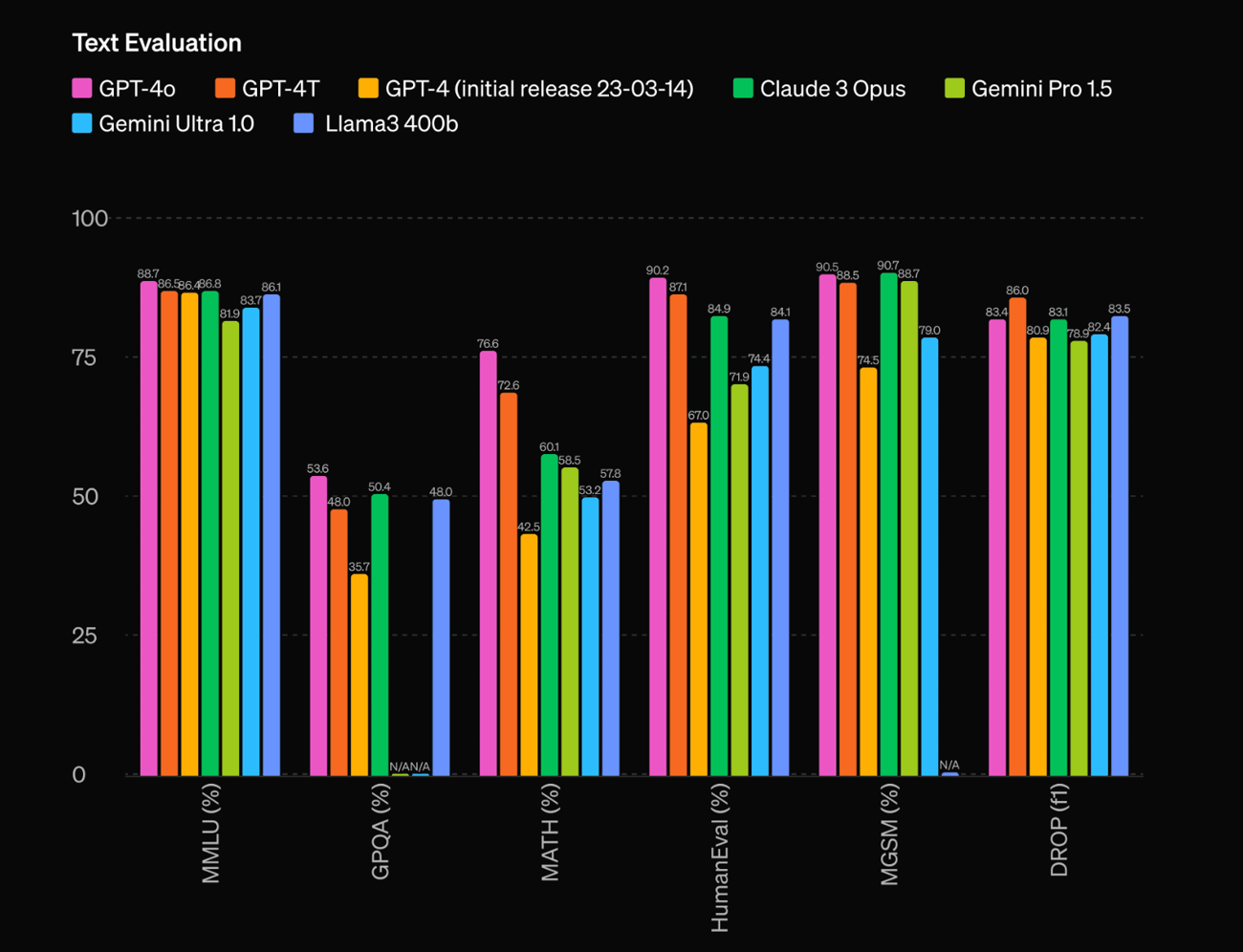 |
The evaluation of GPT-4o, the latest model from OpenAI, shows significant advancements across multiple metrics:
MMLU Benchmark:
- Achieved 88.7% in zero-shot CoT (Chain of Thought) settings.
- Scored 87.2% in the traditional 5-shot no-CoT MMLU.
Multilingual Understanding:
- Surpasses previous models on the M3Exam benchmark with scores of 84.5% in multilingual text and 81.7% in vision evaluations.
Audio Metrics:
- ASR (Automatic Speech Recognition) performance: 3.2% word error rate improvement over Whisper-v3.
- Speech translation: Achieved a BLEU score of 48.6, surpassing Whisper-v3’s score of 45.3.
Visual Perception:
- Achieved 92.4% on the MMMU benchmark, 89.3% on MathVista, and 91.1% on ChartQA.
|
|
Access |
GPT4-o will roll out today for all ChatGPT users through the Web UI, even for free tier users. It is also be available through the API, and the playground.
|
Why it Matters |
The intelligence of AI models depends not only on their reasoning capabilities but also on their ability to understand context and tone emotionally. New user experiences that enable more natural conversations—in terms of speed, emotional and contextual intelligence, and support for real-time information—are a significant step toward achieving Artificial General Intelligence.
This development is especially noteworthy as rumors circulate about a potential deal between Apple and OpenAI. The next version of Siri might be powered by OpenAI.
The intelligence of AI models depends not only on their reasoning capabilities but also on their ability to understand context and tone emotionally. New user experiences that enable more natural conversations—in terms of speed, emotional and contextual intelligence, and support for real-time information—are a significant step toward achieving Artificial General Intelligence.
This development is especially noteworthy as rumors circulate about a potential deal between Apple and OpenAI. The next version of Siri might be powered by OpenAI.
|
Community Feedback |
sama
The new voice (and video) mode is the best compute interface I’ve ever used. It feels like AI from the movies; and it’s still a bit surprising to me that it’s real. Getting to human-level response times and expressiveness turns out to be a big change
|
Itamar Golan 🤓 GPT-4o < GPT-4 It might be more efficient, cheaper, faster, and so on. Nonetheless, I just tested it on a 980 super difficult samples dataset I had on reasoning, coding, math, etc. And it was hitting much less than GPT-4. GPT-4 answers correctly on around ~800 and GPT-4o on ~640. This is a 20% degradation. So again, multi-modalities are cool, latency is awesome, but it ain't smarter.
|
Read the announcement here
|
TRY GPT- 4o
|
|
|
|
|
Webinar: How to Stop Hallucinations in Real Time |
Hallucination detection alone isn’t enough; enterprise AI teams need to proactively intercept hallucinations before they reach end users
Galileo Protect is an advanced GenAI firewall that intercepts hallucinations, prompt attacks, security threats, and more in real-time!
Register to the upcoming webinar to see Protect live in action and learn:
- How the firewall works, including research-backed metrics
- The key features of Protect
- What makes it the first-of-its-kind
Can’t attend live? All registrants will receive an on-demand recording.
|
REGISTER NOW (FREE)
|
partner with us →
|
|
|
|
TRENDING SIGNALS
|
Industry
|
|
⇧ 579 Likes
|
|
Language Models
|
|
⇧ 883 Likes
|
|
Time Series
|
|
⇧ 1672 Likes
|
|
Open Source
|
|
⇧ 250 Likes
|
|
|
|
|
|
|
TOP PAPERS
|
Model Training
|
|
⇧ 4517
|
Problem: Large language models suffer from "under-trained" tokens because the tokenizer is trained independently. These tokens, rarely encountered during model training, lead to hallucinations and degraded performance.
Solution: The paper suggests three solutions: analyzing the tokenizer's vocabulary and its encoding/decoding behavior, detecting "untrained" token candidates using metrics like the similarity between the embedding matrix and the final model layer, and verifying these candidates by iteratively prompting the model.
Results: The approach revealed 0.1–1% of tokens in LLM vocabularies are severely under-trained. The study, applied to models like LLaMA3 and Pythia, uncovered thousands of these tokens. Key insights gained praise from experts, including Karpathy. These findings highlight significant reliability and performance impacts in LLMs.
|
|
Fine-Tuning
|
|
⇧ 1112
|
Problem: LLMs hallucinate when fine-tuned with new factual knowledge, as they learn new information slower than consistent knowledge, increasing the tendency to produce factually incorrect responses.
Solution: The paper proposes SliCK (Sampling-based Categorization of Knowledge), categorizing fine-tuning examples into HighlyKnown, MaybeKnown, WeaklyKnown, and Unknown. Fine-tuning on datasets with varying Unknown ratios helps assess performance and mitigate hallucinations.
Results: LLMs struggle with Unknown examples, leading to a linear increase in hallucinations. Early stopping or filtering Unknowns mitigates performance loss. Metrics: models learn new facts 50% slower and show a 30% increase in hallucinations.
|
|
Language Models Architecture
|
|
⇧ 793
|
Problem: Large language models require substantial KV cache memory, which restricts the handling of long input sequences and deployment on GPUs with limited memory.
Solution: YOCO introduces a decoder-decoder architecture, caching key-value pairs once. The self-decoder produces global KV caches using efficient self-attention mechanisms, while the cross-decoder reuses these caches, reducing memory demands.
Results: YOCO cuts GPU memory usage by up to 10 times, decreases prefill latency from 180s to under 6s for 512K tokens, and improves throughput significantly. It scales efficiently with increased model sizes, handles up to 1M token contexts with near-perfect needle retrieval accuracy.
|
|
|
|
|
|
|
|